Listen to this article:
(This article was written by humans.)
November 30, 2022 was a watershed moment for computer science. That day OpenAI launched ChatGPT, a chatbot that automated a range of human linguistic abilities in a simple, streamlined package. It was most of the public’s very first encounter with generative artificial intelligence (AI) and large language models (LLMs), and it’s an understatement to say that, in layman's terms, minds were blown. For life science leaders, it sparked a stream of powerful questions about the potential benefits such technologies could bring to scientific research and discovery.
In the field of life sciences, chatbots can perform as archivist, guide and co-pilot to scientists and researchers. They can help navigate endless pools of data, develop novel therapeutics, compile drug documentation and protocols and much more. LLM-powered chatbots can retrieve specific, detailed information from vast knowledge corpuses and analyze and categorize it in mere seconds, helping life science companies achieve real-world breakthroughs that will increase efficiency, decrease cost, and most importantly, improve patient care.
Setting up a secure and accurate LLM-based chatbot on proprietary data requires surprisingly little time. This is the reason behind the current proliferation of dedicated LLM-based chatbots across many sectors, especially life sciences.
Recent examples include Microsoft’s BioGPT, a language model for biomedical text generation and mining, Google’s Med-PaLM2, a chatbot that answers medical questions, and Glass AI, a chatbot that interprets unstructured medical data to aid practitioners in their diagnoses. From assisting scientists with routine tasks and learning from their interactions to becoming hubs for knowledge management, and even providing original solutions to existing problems, chatbots can be powerful assets for any life science company and a logical first step for life science leaders to integrate LLMs and AI into their workflows.
Before leaders from biotechnology or pharmaceuticals can reap the benefits of integrating LLMs, they need to put the right technological foundation in place. Doing so comes down to addressing two limitations: privacy and accuracy.
HIPAA-Compliant Chat
Using OpenAI’s API, or any API to leverage an external LLM, starts a data exchange. That means that proprietary and potentially sensitive data move from the user’s premises to those of the owner of the API. This transfer would make many life science and healthcare companies non-compliant with sector regulations such as HIPAA. (Note: Two days prior to the publication of this article, OpenAI unveiled ChatGPT Enterprise. At this point, it remains unclear whether this enterprise-grade version will be HIPAA compliant.)
To remain compliant, any sensitive data needs to remain on company premises, whether physically or in the cloud. By hosting their foundation models on HIPAA-compliant, secure cloud platforms like AWS, and leveraging services like AWS CloudTrail and Amazon GuardDuty, companies in the healthcare and life sciences fields avoid moving or sharing their highly sensitive data. In addition, some open-source models have shown better performances than OpenAI in retrieving relevant data to augment LLMs, a fundamental part of LLM applications. These open-source models are usually adapted, trained and securely deployed on the client’s own cloud environment on AWS.
Loka has been working on machine learning models and generative AI for nearly a decade. We’ve designed HIPAA-compliant solutions, including foundation models and large language models, for life science and healthcare customers. In addition to clearing privacy concerns, our long-standing expertise has proven particularly useful in building accuracy in AI solutions.
Building Laser-Focused Accuracy in LLMs
Ensuring privacy comes down to choosing the right framework to build and host LLM-based chatbots, but setting them up for accuracy is a different matter. ChatGPT “hallucinates,” generating content that is factually incorrect or even completely off-topic. While these might be mere inconveniences for some businesses, for life science companies they would be at best expensive mistakes, at worst life-threatening failures. In fact, a recent paper in The American Journal of Medicine has warned biomedical researchers against hallucinations in ChatGPT.
Sometimes these mistakes are due to the model being trained on unvetted data, such as internet content in ChatGPT’s case. When correctly structured and fed with accurate scientific data, chatbots deliver great accuracy, becoming excellent entry points to leverage the power of AI in life sciences.
“LLMs are great at understanding natural language, yet they don't know everything,” says Telmo Felgueira, Senior Machine Learning Engineer at Loka. “But if we ground them with data, they are really good at interpreting it, providing references to substantiate their answers, even stating that they don’t know, if that’s the case.” This data grounding is usually done by using an underlying, secondary LLM to retrieve the data for a particular question, from which the primary LLM generates an answer.
Plainly put, these secondary language models are built to understand the semantic and contextual meaning of natural language. Their comprehension is based on embeddings, numerical representations of meaning that represent and capture relationships between words and concepts. Different models use different embedding techniques, which in turn influence results.
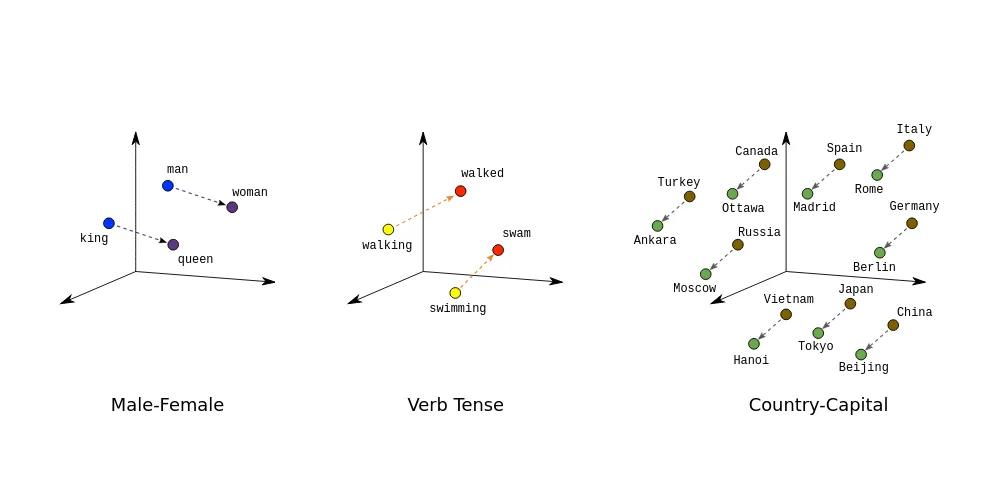
“The better the embedding model, the more relevant the documents that are retrieved by the LLM,” Felgueira says. “When it comes to text generation, OpenAI’s GPT-4 is without a doubt the best model out there today. But in terms of embedding performance, open-source models are by far superior.”
OpenAI’s GPT-4 is without a doubt the best model out there today. But in terms of embedding performance, open-source models are by far superior.
For these reasons, using the right model and providing relevant, structured data to LLM-based chatbots are key to obtaining accurate results, which is especially crucial in life sciences, where there’s little to no margin for error.
Organizing Unstructured Data and Searching Complex Semantics
With the right basis in place to ensure privacy and accuracy, the natural next step in building an LLM-based chatbot is focusing on the data sources it will tap into. Life science companies typically need LLMs to work with a variety of structured and unstructured internal data: text-based files in different formats, numerical values, databases, even images. Examples include but are not limited to scientific papers, lab reports, clinical trial results, spreadsheets and images.
Several companies are tapping into the capabilities of LLMs to perform accurate semantic searches across vast bodies of text-based knowledge, which become central knowledge repositories. For example, an LLM-powered chatbot can conduct complex searches across a large set of information, bringing up all relevant results based on the actual meaning of the question, not the specific keywords it contained. In the same way, the chatbot could comb through all the proprietary information on the development of a new drug, including clinical trials, to retrieve a specific piece of information. These are typical use case scenarios that can save researchers and scientists a tremendous amount of time and energy, freeing up human resources for key decision-making tasks.
Using LLMs to Categorize, Rearrange and Reanalyze Data
Once an LLM understands unstructured and structured data, it can quickly and easily categorize it, reanalyze and rearrange it in as many ways as needed. This enables life sciences companies to leverage the data they already own to gain new insights, conduct statistical analysis and compare results across large volumes of documentation.
This way, researchers can avoid performing repetitive tasks or overlooking important information, which will in turn helps businesses better channel their resources. Typical use cases would be to ask an LLM-based chatbot to pull up all drug candidates from literature for a specific disease the company has been studying. Or to answer business-specific questions in natural language, such as comparing the starting conditions of two clinical trials. Or to find information on research methodologies.
Extracting Further Value
LLM-based chatbots can do much more than answer even the most complex questions. Some groundbreaking use cases include text summarization, text generation and image captioning.
In this sense, scientists could ask a chatbot to summarize key findings from proprietary information, or condense lengthy drug trial reports into compact overviews. The LLM-based chatbots could also help with key tasks such as creating descriptions of complex bioengineering processes, producing informative captions for drug mechanism visuals,and even creating summaries of drug indications and contraindications. This is exciting new territory for scientists and researchers, as it allows them to complete long and complex tasks quickly and easily.

What’s Next?
The capabilities of LLMs in life sciences are increasing exponentially. Every day, new possibilities open up for companies to rely on powerful, customized and privacy-compliant tools to save time and money and advance science at the same time. So what lies ahead for LLM-based chatbots?
From a technical standpoint, we can expect new developments in the interactions between language models and external applications, so-called agents. “Based on the user’s specific question, LLMs could decide on a case-by-case basis to interact with external tools, such as interrogating an external database, to offer more complete answers,” Felgueira says.
But given the right data set, LLMs can go well beyond leveraging existing assets. LLMs can provide creative solutions to specific problems.
"For example, if we were to provide one with a set of data about proteins, we could ask it to generate entirely new proteins from scratch with the same characteristics as those of the training set,” says Jorge Sampaio, Bioinformatics Engineer at Loka. “Despite having never been seen before in nature, these proteins would still adhere to nature's principles and be as functional and stable as the natural ones. This so-called de novo protein design constitutes an extremely exciting use case for drug development.”
We could ask it to generate entirely new proteins from scratch with the same characteristics as those of the training set.
The benefits of LLMs are incredibly practical, they’re potentially massive–and they’re available for the taking. The key is to find a roadway in, and to engage in a safe, secure way, especially when the stakes are as high as in life sciences. The time is now for companies to integrate these powerful tools in their daily business.
Loka partners with companies across the life sciences data journey, especially in that crucial first step. We even created our own chatbot, Clementine, that acts as a blueprint for customers to create their own AI-powered tool. Connect with us to discover how to integrate LLMs into your workflows and learn more about our services.